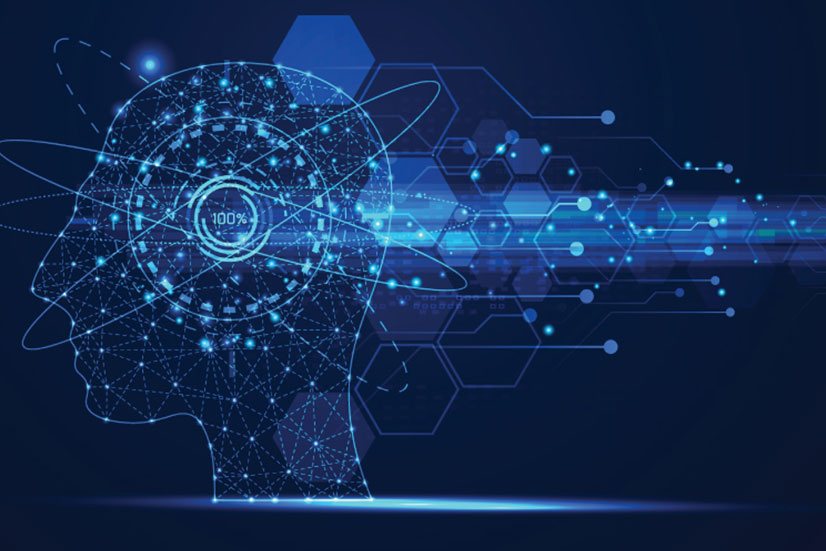
MLOps in the Age of Generative AI: Charting a Course for Success
In the rapidly evolving technological landscape, Artificial Intelligence (AI) stands as a testament to innovation and transformation. Recent years have witnessed a paradigm shift, primarily attributed to the remarkable progress in Large Language Models (LLMs) and Generative AI. These advancements, astonishing even to the most dedicated technology enthusiasts, have unlocked a new realm of possibilities across industries. As leaders in this dynamic era, we must recognize the significance of these breakthroughs and adapt our strategies to harness their potential.
The proliferation of applications driven by Generative AI underscores the critical need for a robust MLOps (Machine Learning Operations) approach. Such an approach is pivotal for effectively automating, scaling, and managing these advanced AI models. Furthermore, as the compliance landscape becomes increasingly intricate, especially within the realm of Generative AI, MLOps becomes indispensable. In a recent Gartner survey, CEOs from diverse industries unanimously identify AI as the most significant disruptor in the current business landscape.
As a Google Cloud partner, our team at Intelliswift Software is deeply engaged with a wide array of tools that constitute the bedrock of MLOps, including:
- Vertex AI: Offering an all-encompassing platform for machine learning projects, Vertex AI guides from data preparation through model construction, training, and selection. It seamlessly deploys models into production and provides monitoring tools for ongoing performance tracking. This comprehensive approach streamlines processes, maintaining effectiveness over time while eliminating the need for tool-switching and boosting efficiency.
- Cloud Dataproc: A managed service streamlining big data frameworks such as Hadoop and Spark for machine learning. It abstracts cluster management complexities, enabling focus on model development and training. Through infrastructure automation, Dataproc empowers data scientists and engineers to harness distributed computing's potential without being burdened by technical complexities.
- Cloud ML Engine: A managed service designed for machine learning workflows, offering a complete toolkit for model training and deployment. It handles data pipelines, preprocessing, distributed training, and real-time API deployment. Centralizing tasks streamlines the process, freeing data scientists to concentrate on model development and deployment instead of infrastructure management.
- Cloud AutoML: By empowering users to craft customized machine learning models without requiring extensive coding expertise, Cloud AutoML streamlines stages ranging from data preprocessing to hyperparameter tuning. This democratization of machine learning extends its benefits to domain experts, enabling them to harness AI for specialized use cases, fostering inclusivity, accelerating AI adoption, and catalyzing innovation.
- Cloud AI Platform Prediction: Facilitating the seamless deployment of trained machine learning models as real-time APIs, Cloud AI Platform Prediction provides a managed environment for accurate predictions. With a focus on accessibility, scalability, and performance, this tool seamlessly integrates machine learning capabilities into applications and services, enhancing their functionality and value proposition.
- Cloud Monitoring: Crucial for maintaining production models, Cloud Monitoring is pivotal in monitoring model health and performance. Equipped with tools that monitor behavior, key metrics, and anomalies, this tool furnishes invaluable real-world insights. These insights enable informed decision-making, optimization of resources, and the enduring effectiveness of machine learning solutions, contributing to sustained success and efficiency.
- Cloud Logging: As a central repository for machine learning logs, Cloud Logging aids in troubleshooting, auditing, and detailed analysis. Systematically organizing logs simplifies error identification and problem diagnosis and offers valuable insights into workflow behavior and performance, enabling continuous improvement and optimization.
- Cloud Artifact Registry: Concentrating on version control and reproducibility within machine learning, this tool provides a dedicated repository for essential artifacts such as trained models and metrics. By ensuring dependable change tracking, facilitating team collaboration, and promoting consistency across diverse iterations of your machine-learning models, Cloud Artifact Registry fosters a well-structured and controlled development environment for sustained success.
- Cloud AI Platform Pipelines: Empowering the end-to-end machine learning workflow automation, Cloud AI Platform Pipelines facilitates the creation of reusable pipelines encompassing data preparation to model deployment. With stages covering preprocessing, training, and evaluation, this tool enhances consistency, reduces errors, and promotes seamless team collaboration. This acceleration of development and deployment processes optimally positions organizations for rapid progress and innovation.
Conclusion
With these tools at our disposal, we can easily create, deploy, and maintain machine learning models at scale while ensuring reliability and efficiency. As the landscape of Generative AI continues to evolve, our unwavering commitment to staying at the forefront of MLOps innovation empowers us to navigate this dynamic and ever-changing terrain confidently and proficiently. By leveraging these strategic tools, we stand poised to embrace the boundless opportunities presented by the Age of Generative AI while upholding the highest standards of operational excellence & compliance.
We, at Intelliswift serve as Google's Preferred Engineering and Strategic Partner, aiming to expedite innovation, employ machine learning and intelligent tools for accomplishing business outcomes, and provide entry to an advanced suite of APIs that facilitate secure growth at an enterprise level. Reach out to our Google Cloud practice today to share best practices and accelerate your rollout of real-world applications.
Sanjay Kalra, VP, Digital Transformation and Head, Alliances
Sanjay is a highly experienced subject matter expert in Information Technology, Product Engineering, Customer Experience, Cloud, Automation, Data Analytics, and AI – which enables him to serve as a trusted advisor to enterprises and growing organizations.